Don’t send your AI adoption down the rabbit hole - Key in 'Data Science'
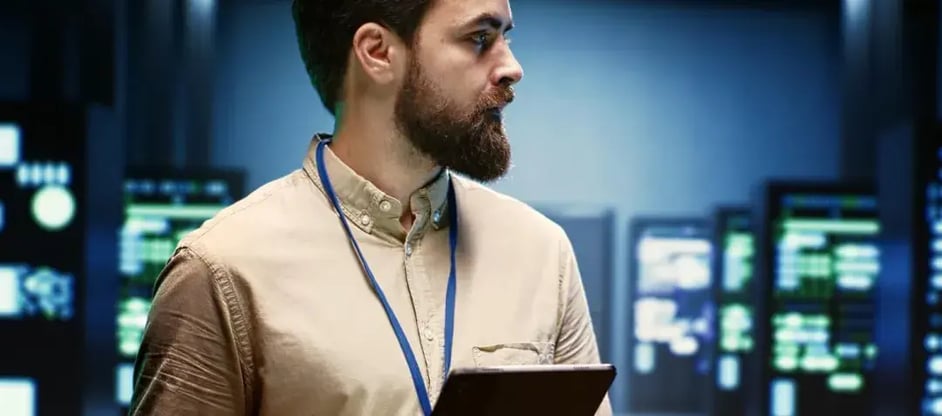
Imagine a company eager to embrace AI, pouring millions into automation and predictive analytics. They launch pilot after pilot—chatbots, fraud detection, supply chain optimization—only to hit a wall when it’s time to scale. Sounds familiar?
This struggle isn’t about AI’s potential; it’s about missing the right foundation. Without strong data science capabilities—quality data, robust models, and skilled teams—AI projects stall, failing to deliver real impact.
Can data science spice up your love affair with AI?
A recent study by the European Banking Authority explored the use of machine learning techniques, such as random forests and neural networks, to automate bank supervision. The study found that while these AI models offered improved methodologies, they still faced limitations due to reliance on historical data, which could not predict unprecedented financial crises.
Highlight:Ensuring data quality and integrity is essential to fully realize AI's potential and drive meaningful impact.
This underscores the critical need for high-quality data and robust data science practices to ensure AI models deliver accurate and unbiased results. Ensuring data quality and integrity is essential to fully realize AI's potential and drive meaningful impact.
So, jumping into AI without data science? Enjoy the free fall.
Garbage in garbage out
Back in 2017, Amazon scrapped its AI hiring tool after realizing it was biased against women. Trained on a decade of resumes—mostly from men—the system started favouring male candidates and even downgraded resumes with words like ‘women's’ or those from all-women colleges. This highlights a major AI pitfall: if your data reflects past biases, your AI will too.
AI models rely on quality data. If data is biased, incomplete, or unstructured, the model will produce flawed insights.
When AI and your business goals ‘Don’t Speak’ the same tongue
AI flops when it’s out of sync with business goals. Imagine a retail company using AI for demand forecasting but not linking it to real-time supply chain data—useless, right? Without the right data strategy, even the smartest AI can miss the mark. The key? Align AI with real business needs, not just trends.
Want to nail AI adoption? Know why data science is the real MVP (Most Valuable Player) and get your hacks in place.
Get your data in shape
Messy data leads to unreliable AI. Ensuring data quality before feeding it into AI models leads to more accurate and reliable outcomes.
Set the ground rules
AI needs clear guidelines on data quality, privacy, and ethics. Establishing strong governance practices helps mitigate risks, ensure compliance, and maintain trust.
Keep AI transparent
AI should provide clear, explainable decisions rather than acting as a black box. Building models with clarity in mind enhances accountability and fosters confidence in AI-driven outcomes.
Improve, always
AI is not a one-time implementation. Continuous monitoring, evaluation, and refinement ensure that models stay relevant, accurate, and aligned with changing business needs.
AI without data science? That’s a glitch waiting to happen!
AI isn’t a silver bullet—it’s only as powerful as the data it learns from and the strategy that guides it. Companies pouring millions into AI without a solid data foundation often hit the same roadblocks: failed pilots, biased decisions, and models that don’t scale.
From Amazon’s hiring fiasco to financial models that crumbled under real-world complexity, the message is clear: garbage data in, garbage results out. Without high-quality data, strong governance, and continuous optimization, AI becomes just another expensive experiment.
So, before you jump on to the AI bandwagon, ask yourself: Are you building on a rock-solid data science foundation—or just hoping for the best?
Partner with our experts to know what you need and define your AI-driven business goals.