Master Data Engineering practices to build powerful data platforms—Here’s How!
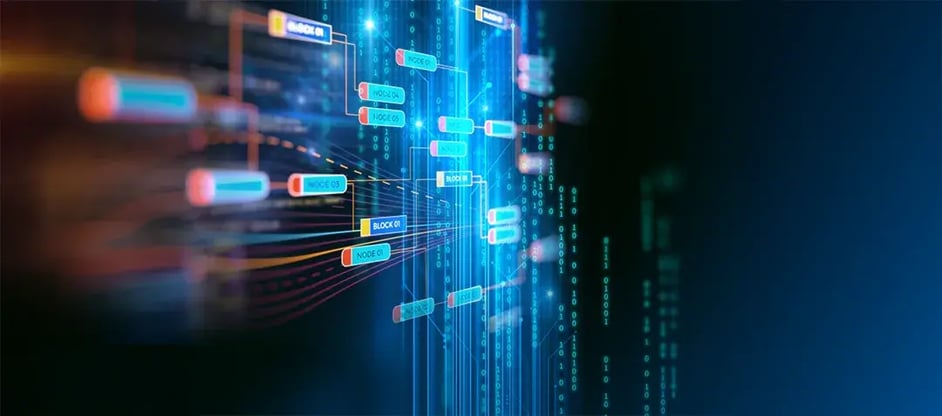
Is there anything like ‘too much data’? Yes, most certainly!
The world is scrambling with overflow of data and so are businesses. At the heart of it, all businesses in the real world are data-driven, as it induces intelligence for growth. Hence, it’s crucial that your data platforms are smart enough for you to process, analyze, extract insights, and make relevant decisions. In a haystack of jumbled up strings, effective and scalable data engineering practices needle out the right information for you to optimize.
Let’s get this right! Data is no longer an IT department concern area; it is a C-suite strategy discussion.
Forrester’s trend report on ‘Evolve Data And Analytics Roles And Skills For The Adaptive Enterprise’ summarizes, “Data-driven businesses must continuously improve five capabilities: application, data, process, people, and infrastructure. Weaknesses and gaps in any one of the competencies — including people — will decrease the chances for success of the entire data-driven business approach.” All in all, building advanced data platforms with effective engineering has never been more critical for better business outcomes.
Pin up this 5-step rule book and make it a win-win deal!
Spend time on a thought-through data architecture: A well-designed data architecture is the foundation of a powerful data platform. It defines how data is collected, stored, processed, and accessed. Choosing the right architecture ensures seamless data integration and efficient analytics. |
Bring scalability with Cloud: As data volumes grow, scalability becomes a critical factor. Cloud platforms like AWS, Azure, and Google Cloud offer managed services for big data processing. Scalable infrastructure allows businesses to handle increasing data loads efficiently. |
Optimizing data for better application: Raw data is rarely usable in its original form. Transforming and enriching data ensures high-quality analytics. Efficient data processing speeds up decision-making and enhances insights. |
Can’t skip governance and security: Data privacy and compliance are critical for building trust and avoiding legal issues. Strong governance policies keep data secure while maintaining transparency. |
Real-time analytics and automation can do wonders: Modern businesses require real-time insights to stay competitive. Real-time dashboards and automated monitoring can prove to be effective for deriving insights to enhance operational efficiency. |
Text highlight: “Data-driven businesses must continuously improve five capabilities: application, data, process, people, and infrastructure.”
Stewart Bond, Vice President of Data Intelligence and Integration research at IDC, shares, "Modern data environments are highly distributed, diverse, dynamic, and dark, complicating data management and analytics as organizations seek to leverage new advancements in generative AI while maintaining control. Innovations in data and analytics technologies over the next five years will help organizations get out of the current AI scramble and into adoption and move forward to become future AI-fuelled businesses." The realm of data will keep evolving, but what you need to do is find peace in this chaos for inducing more intelligence and automation into your ecosystem.
Effective data engineering practices form the backbone of data analysis and data science by structuring, preparing, and ensuring its accessibility. Going by industry case points, in ecommerce, it involves cleaning and organizing customer transaction data to improve personalized recommendations. In healthcare, data pipelines aggregate patient records from various sources, enabling accurate predictive analytics for better treatment plans.
Does this sound compelling for your business growth? Get this into perspective, the idea of engineering your data streams is as good as it’s implementation. If done right, it can empower you to derive meaningful insights and make smart decisions.
Work with us to experience the impact of good data.